Enterprise Intelligence and Synthetic Intelligence have all the time belonged collectively. However as BI has grown a bit inflexible, AI has taken the largest leap but with the arrival of LLMs, says Avi Perez of Pyramid Analytics. Now, non-tech customers can use the complete energy of knowledge and take productiveness to new heights. There are challenges nonetheless, like knowledge high quality or privateness, however Avi discusses options and warns NOT to overlook that practice.
The CTO vs Standing Quo collection research how CTOs problem the present state of affairs at their firm to push it towards a brand new top … or to reserve it from doom.
“We’re at an inflection level now […] you may be lacking one thing dramatic.”
In concept, it’s simple to grasp why Synthetic Intelligence may very well be a recreation changer for Enterprise Intelligence. As an alternative of constructing charts or painstakingly question knowledge, your customers may simply ask questions verbally and get the insights they want in response.
“As if it really works like that!” you ask in disbelief. Nicely, you aren’t completely improper, however plainly this superb of an AI-driven BI that serves as an clever advisor moderately than only a assortment of pie charts is nearer than chances are you’ll assume.
Avi Perez is engaged on it as we converse, and he advised us how he and different gamers available in the market deal with challenges similar to:
- what LLM to decide on,
- combining a generic LLM with a customized overlay vs coaching the LLM itself,
- knowledge privateness – utilizing LLMs with out sending any delicate enterprise knowledge to them,
- tailoring the LLM answer to an organization’s distinctive wants,
- measuring the impression of AI-driven BI.
Sounds attention-grabbing? Meet Avi and discover out what the way forward for BI holds!
About Avi & Pyramid Analytics
On-line analytics knowledgeable, a co-founder and CTO of Pyramid Analytics, at its helm since 2008. Avi’s imaginative and prescient drives the event of Pyramid’s enterprise analytics options. What’s extra, his deep curiosity in knowledge science and AI is the spine of the corporate’s R&D. Previous to his work for Pyramid, he co-founded and managed a U.S-based healthcare analytics firm, Urix, personally architecting its analytics techniques.
Knowledge analytics, embedded analytics, knowledge science, company technique, Synthetic Intelligence
Launched in 2008, with places of work in Amsterdam, Tel Aviv, and London, amongst others, Pyramid provides enterprises an end-to-end embedded analytics answer that features knowledge prep, enterprise analytics, and knowledge science. Gartner® Magic Quadrant™named Pyramid one of many “visionaries” of the ABI market, partially due to its gen AI capabilities. In Could 2022, the corporate secured $120 million in funding at a virtually $1 billion valuation.
Pyramid Analytics in 2024
Hiya Avi, How are you doing as we speak? I do know that Pyramid Analytics has been doing properly not too long ago. You’ve shared a screenshot from Tremendous Knowledge Brothers that exhibits Pyramid is the fastest-growing embedded analytics answer. However chances are you’ll be much more proud that Pyramid Analytics has been featured in Gartner’s Magic Quadrant for Analytics and Enterprise Intelligence (ABI) Platforms report.
How good is 2024 for you?
We had a spectacular yr, being named a visionary within the Gartner Magic Quadrant for Analytics and Enterprise Intelligence (ABI) Platforms. We’re on par with Tableau and only a bit off from Microsoft.
It’s exhausting to go in opposition to behemoths like Microsoft, SAP, Oracle, Google, or AWS. However you may compete with them on a product degree, and also you don’t essentially have to be a behemoth to construct the perfect product available in the market.
The Magic Quadrant evaluations the entire firm, together with the product. However our most essential achievement is being included within the Essential Capabilities report, which is a sister report back to the MQ and is only a evaluation of the product. In that one, we’re clearly the primary ranked answer based mostly on function and performance – we scored in each capabilities after which throughout all of the totally different use instances.
You’ve been with Pyramid for 16 years. Did you anticipate that it might turn out to be one of many main BI platforms on the planet again in 2007
We didn’t anticipate to be the place we at the moment are. Our product hasn’t modified a lot shortly, but it surely takes numerous muscle and cash to get the message on the market and educate {the marketplace} and analysts – it’s one of many handicaps of being a small firm.
The reality is that if I stripped the answer we had in 2007 to its necessities, you’d be shocked to seek out out it’d be roughly the identical sketch as what we now have as we speak. It goes again even additional. In 2001, my earlier firm, a vertically centered healthcare firm within the US, tried to deal with what Pyramid is fixing as we speak.
The Pyramid idea has been round for a very long time, however solely now have all of the items come into play. Everybody acknowledges all the weather the way in which we understood them 25 years in the past. You could possibly say that the analysts caught up with us. Our impression of the place enterprise analytics was going was proper all alongside.
An enormous a part of the puzzle is AI and what it has delivered to the BI market. Gartner’s report confirmed that we’re clearly one of many leaders in gen AI integration into BI.
Are you able to inform me extra about it? What’s so particular about the way in which you deal with gen AI?
Positive, however first, we have to take a step again.
AI has really been part of BI for a very long time. It’s one of many extra apparent locations to stay it in. You need to show the BI performance to many customers, however numerous what you present is difficult. You want all these magical instruments to unravel issues. We name it AI. Plenty of gamers in the marketplace have roughly of it.
Pyramid Analytics has tons of AI. Now we have dozens of distinctive AI constructs and parts all through the platform which are AI-centric. Each one among them has thousands and thousands of various elements.
The gen AI framework is comparatively new, to the extent that it goes hand in hand with giant language fashions (LLMs) and the deep neural community, which has come to the final consideration within the final two years with ChatGPT and OpenAI.
The interpretive energy of LLMs is in a special league than what was earlier than. Its profound functionality to grasp what the consumer says and to reply intelligently is unbelievable. That is positively a quantum leap. It’s pivotal in BI. We need to let folks faucet into the sophistication of analytics, however the overwhelming majority of customers are non-technical and don’t know the nuances of what to ask and the way to ask it. The LLM bridges the hole.
Having stated that, Pyramid’s gen AI performance is predicated on a mannequin the place we outsource to the LLM solely what we name recipe making. We don’t outsource the evaluation and question. Pyramid handles the baking of the recipe, and the outcomes are handed to the consumer.
It’s the symbiosis between the 2 applied sciences that produces a really refined, unbelievably intelligent answer. The mannequin is extremely scalable and doesn’t require you to pump all of your knowledge into the LLM or to fine-tune the LLM. You’ll be able to take any generic LLM, and in concept, it’ll work.
And most significantly, you don’t must share your knowledge with the LLM. Huge corporations with their top-secret mission-critical company knowledge aren’t thrilled about sending it to OpenAI.
I feel it’s a template that every one distributors will use as a result of the concept that you may fine-tune an LLM by yourself in a approach that permits you to construct one thing distinctive with the information is slightly fictitious in the intervening time because it stands as we speak.
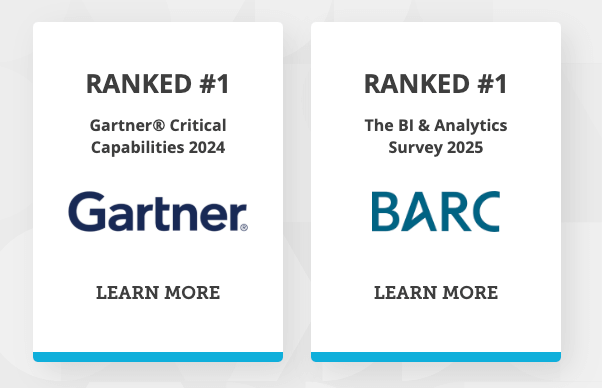
Synthetic intelligence – it takes ever extra to face out
AI is a giant a part of what I wished to speak to you about as we speak. Earlier than we get to the principle level, let’s speak about AI itself for some time. In fact, everybody does (or desires to do) that as we speak, however one among my earlier visitors advised me that many corporations nonetheless don’t perceive simply how virtually helpful AI has turn out to be for companies in a really brief time. What do you say to that?
I can’t stress it sufficient, so I’ll repeat – the interpretive potential to grasp language on the way in which in or to simply accept it from the consumer and to reply intelligently on the way in which out is a dramatic quantum leap from what got here earlier than it. That solves one of many nice limitations to AI deployment – the interface.
Having stated that, gen AI nonetheless has methods to go, particularly within the BI area. There are important challenges to unravel earlier than I can truthfully let you know that gen AI will begin to displace conventional BI constructs fully.
Everybody thinks that the top purpose of enterprise intelligence and analytics is the pie chart. It’s not. That is only a means to an finish. The top purpose of analytics is to have a look at the information and decide. What the consumer really desires is to listen to: “You don’t want to have a look at the pie chart anymore. Right here’s the choice you search for”.
I all the time inform folks the analogy about Captain Kirk from Star Trek. He by no means used any type of software program to program or direct his machine the way to fly. He simply stated: “Enterprise, fly me from right here to right here!” He may care much less the way you get there. Think about that I may discuss to the machine and say: “Don’t draw me a pie chart of my gross sales so I can work out what I ought to promote tomorrow. As an alternative, simply inform me what I ought to promote tomorrow.”
We’re already near that in some industries; assume GPS or mapping instruments. You sort within the handle and inform the automobile to drive. In fact, you continue to must drive, however what we’d actually love to do is sit again and inform the automobile to drive us.
Self-driving vehicles might turn out to be commonplace quickly, isn’t that proper?
Sure. It’s imminent. And I would like the identical for enterprise decision-making.
I would like my software program to inform me what I must do tomorrow to be extra worthwhile. And the following step from there could also be: you do the factor that can make me extra worthwhile. You plug your self into all of the meeting strains, activate the machines, and determine which vehicles to make or which chairs. Within the meantime, I’m going to be on the seashore.
Gen AI clearly has numerous potential, however there are additionally some limitations. On the very least, they exist at this second.
Sure. The interpretive functionality, which I talked about, is simply step one. The second step, the evaluation, continues to be type of awful.
The LLMs aren’t designed for doing arithmetic. For those who look on the web, you’ll discover that there’s numerous curiosity in constructing deep neural networks that clear up mathematical deterministic-type responses. I feel it’ll occur ultimately. And it’s going to be a dramatic change.
On prime of that, there’s a headache about utilizing present reside knowledge with LLMs. These fashions are skilled on knowledge. Whenever you ask ChatGPT what the price of pizza is on Madison fifth in New York, the reply is predicated on some knowledge factors it discovered on the web three years in the past. Even when they’ll discover a option to get some more energizing knowledge, their interpretation of info is predicated on what it was skilled on.
However my company knowledge is just not sitting on a web site. It comes from a company structured database. The LLM that we perceive as we speak is just not ready to interpret all this knowledge, which is like two billion rows or 5 petabytes sitting on Databricks or Snowflake.
This is likely one of the issues that Pyramid solves as we speak. It’s a very attention-grabbing approach for gluing LLM’s potential to interpret phrases with Pyramid’s potential to interpret knowledge. We proceed to make it act an increasing number of intelligently with out having to maneuver the information into the LLM, which is, because it stands as we speak, someplace between fictitious and impractical.
You talked about makes an attempt to make LLMs clear up math issues. What are another AI tendencies you might be notably inquisitive about as of late?
I can let you know in regards to the challenges we’re making an attempt to unravel within the BI and AI area as we speak. They’re all about discovering methods to ask extra highly effective questions and get extra correct and particular solutions about company knowledge.
The primary group of initiatives pertains to with the ability to question your personal company knowledge and mix fragments of it into the LLM. The mannequin ought to mix the information fragments, my query, and its deep neural community studying functionality to provide me an clever reply.
Think about an accounting system. It doesn’t have paragraphs of textual content explaining revenue and loss. It’s a giant database with tables. One desk is for the account, one other desk is for dates, and one other one is for price facilities or enterprise items. The final desk is the very fact desk, which has all of the numbers in it. There isn’t a lot textual content to it. There are numerous small items of data that collectively construct a narrative, however they’re type of bland on their very own.
Gen AI is nice at dealing with textual content – RAG (Retrieval-augmented Technology) processes document-type knowledge shops, fragments of textual content and paragraphs, and something text-based. Nevertheless, it doesn’t work properly for tabularized knowledge like that of the accounting system. The issue is that the overwhelming majority of company selections are made on such knowledge. Tables include your monetary knowledge or a giant a part of your CRM knowledge.
We’re engaged on translating RAG into one thing that works properly with tabularized knowledge. It typically boils all the way down to auto-vectorization. We’ve obtained working prototypes, and they’re able to some unbelievable issues. However we nonetheless want to determine if we will make it work at a scale.
The opposite group is about with the ability to create formulation. I’m not speaking about easy issues, similar to: “sum up my gross sales figures.” We’re engaged on introducing actual company equations which have an idea behind them that you just shouldn’t have to elucidate, similar to: “Present me my gross margin proportion yr on yr by buyer.”
The mannequin wants to grasp what the gross margin is and who defines it. What’s extra, it wants to grasp what yr a given piece of knowledge belongs to. It might be simple for people to do, however for the LLM, it’s a special story.
However we’re getting there. We’re nearer and nearer to a world through which we will ask our software program straight the way to make our store extra worthwhile. It’s a profound concept. Whoever will get there first goes to construct one thing phenomenal.
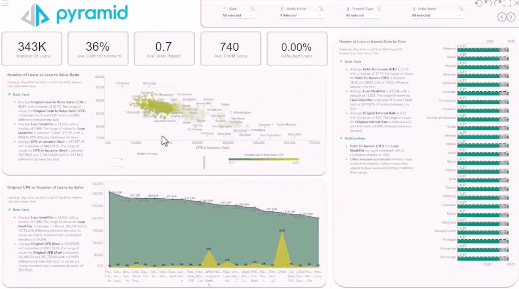
AI-powered Enterprise Intelligence – the dialog
Let’s get to Enterprise Intelligence. In fact, BI is a really outdated self-discipline, however AI has launched numerous recent air and innovation to the sphere. One among our latest visitors stated that builders adapt companies to frameworks, however AI adapts itself to what you are promoting. Within the BI context, one may say that with AI, as a substitute of studying from the previous, you expect the long run.
What are probably the most important variations between a conventional BI answer and an AI-driven one, in your opinion?
If I’m going again 30 years to the Nineteen Nineties, which feels like an eternity, BI was about constructing stories or visualizations. It was designed by builders who handed stories to finish customers, who had been primarily enterprise folks. Builders emailed the report, or worse, they printed it out and handed it on a chunk of paper.
Within the 2005-2010 period, there was a giant leap towards self-service. The instruments grew to become really easy to make use of that enterprise customers may construct their very own stories, slicing and dicing them as they happy. This required extra growth, but it surely gave companies extra energy. It was simpler to think about an concept and implement it on the spot moderately than sequence a venture, rent the assets, after which wait three months for that to be applied.
Round 2015, BI prolonged into the information area. It was the period of knowledge preparation. Folks began spending time cleansing or fixing knowledge, interacting with the semantic layer, and so forth. This was good as a result of good knowledge is an effective precursor to high quality knowledge evaluation.
Nevertheless, no one actually desires the developer to current them with a wonderfully curated knowledge warehouse. They need to construct their very own one or use their very own spreadsheet.
Within the years 2015-2020, you possibly can observe additional merging of BI and knowledge evaluation after which a real rise of knowledge science with all of the tons of data and billions of rows. The issue was that it was all very developer-centric and scientific. An extraordinary Joe couldn’t play with these items.
The massive problem was to make it extra user-friendly. We’re nonetheless in the midst of that cycle. Someplace down the highway, AI has turn out to be the focal point as a option to simplify numerous the sophistication in knowledge science and knowledge prep.
In its newest incarnation, round 2021-2022, Gen AI introduced a quantum leap in interpretive functionality. Out of the blue, folks realized that they might do numerous issues rather more simply than ever earlier than.
In concept, AI guarantees to unleash much more of that cleverness and class that permits much less technical folks to do knowledge science. We’re not there but, however we’re getting nearer.
Enterprise folks would be the solely ones to learn from this. Would you agree that gen AI-driven BI additionally will increase the significance of the CTO in BI-related decision-making? AI is, at its core, a really technical topic, and CTOs would be the ones implementing it.
Positively, however there’s nonetheless numerous confusion, even amongst CTOs.
The CTOs of our clients typically inform me that they’ll’t wait to implement ChatGPT on their web site to reply the shopper’s questions. However LLM itself doesn’t have the solutions. The LLM can act because the interpretive bridge to get you solutions. However until you’ve particularly skilled it in your particular enterprise downside, it doesn’t know something about it.
Take a giant firm like Financial institution of America – ChatGPT has no concept the way to clear up financial savings account points for its customers. Maybe it may get some content material on the way to clear up financial savings account points for patrons from 50 totally different banking websites, however that will solely produce a generic reply.
Even worse, it might fully hallucinate, as it’s generally referred to. It offers you a selected reply, however it’s not in any respect how Financial institution of America actually solves the account points. Each financial institution does it slightly bit otherwise.
Nevertheless, it might be rather more helpful and useful if I may immediate the LLM to ask me what sort of response it wants to provide after which produce a response distinctive to me.
Perhaps massive corporations can attempt to retrain basic fashions based mostly on their very own particular company necessities. But when you understand one thing about coaching LLMs, you notice how fictitious it’s as we speak. Lots of people have slowly understood that fine-tuning LLMs is slightly over-sold. It’s not going to go the way in which you assume it’s.
For this reason I imagine the Pyramid mannequin will win within the foreseeable future. However there’s nonetheless a problem right here – we’re handing you a generic answer that’s untrained in your knowledge, enterprise instances, language, and nomenclature. We have to make it work with something.
This confusion is just not the one factor that will maintain CTOs again from utilizing gen AI-driven BI. For instance, they might have a really massive legacy system and aren’t fast to innovate. The CTO took an curiosity in AI and desires to attempt it. How would they start a dialog and acquire assist for one thing like this?
Let’s say that you just’ve invested in what’s as we speak a legacy BI platform – it might have been developed 30 years in the past and hasn’t actually saved up with the instances. There’s no AI performance. Chances are you’ll watch for some AI options to be added, however it might by no means occur.
Sadly, it’s fairly clear that these legacy distributors aren’t going to put money into AI. It’s too troublesome to retrofit it into their system. For those who take a look at the platforms that had been constructed round 25-30 years in the past, you may see that they both don’t have any gen AI or it’s very gentle.
Whenever you use a legacy BI platform, chances are you’ll ponder side-by-side deployment – one for the outdated world and one for the brand new world. However I might argue that sooner or later, you must transfer on. Folks typically say to me, “Wow, that sounds drastic.” I ask them if they’re nonetheless working round with a Nokia flip cellphone or engaged on an i386 chipset. In the event that they use fashionable applied sciences, perhaps it’s time to attempt the identical method with analytics.
In addition to, legacy options, by definition, are supposed to be modified. Relying on which answer you’re taking, that change mannequin might be dramatic or not.
Essentially the most troublesome half to alter is the combination on the information facet. I’ll use an instance of how we did that at Pyramid Analytics.
Pyramid focuses on what we name direct querying ideas. You are taking Pyramid, level it at your present knowledge state (may very well be in an information lake, database, or knowledge warehouse), and we work on it instantly. So, to make use of Pyramid’s gen AI assemble, you first set up the platform, which takes lower than an hour, and level at your present knowledge property. 5 seconds later, gen AI works out of the field.
Now we have a singular focus in the marketplace. We don’t copy your knowledge or ask you to restructure it to suit how we have to question it. Time-to-result with Pyramid is measured in hours, and the result’s pretty much as good as you’ve seen in any demo we’ve given. Another corporations are engaged on variations of that mannequin, too. Others require you to make dramatic adjustments to your knowledge technique or ditch it as a result of they won’t work with it in any respect.
The rationale why not having to compromise your knowledge technique is so essential is that the tail shouldn’t wag the canine. On this case, your analytics answer and gen AI shouldn’t dictate your knowledge lake technique. It ought to work to enhance it.
Any CTO as we speak must determine the place they need to land on that continuum. In my private expertise, it’s higher to step by step enhance moderately than retool, reskill, rebuild, or reinvest. In any other case, what may very well be a five-hour train turns right into a five-year story. That’s why I like to recommend selecting an answer that retains up with the instances. Then, concentrate on enhancing your integration with it. In any other case, chances are you’ll be overlooked of the gen AI revolution.
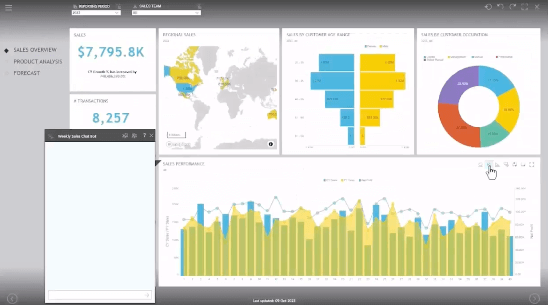
AI-powered Enterprise Intelligence – the implementation
Let’s transfer on to the implementation. A CTO has satisfied the stakeholders and is getting ready to advise on the technical implementation of an AI-driven BI answer. What issues ought to they deal with?
The primary query can be: which LLMs do I need to use? Pyramid helps a number of LLMs, and you may select to make use of greater than one after the other.
When selecting an LLM, you must contemplate the price. There’s a particular pricing differential between GPT-4, the Azure AI suite, Google Gemini Professional suite, Claude, Mistral, or every other answer.
In my expertise to this point, OpenAI’s GPT-4 suite produces the highest-quality outcomes for what we do with it in our testing. It’s additionally probably the most costly of the lot, and so is Claude. Mistral and Google Gemini run for much less, however the high quality is a number of proportion factors decrease. There are trade-offs there.
Languages are additionally an issue. OpenAI helps a number of localizations. You’ll be able to converse to it in German in opposition to an English knowledge set, and it’ll reply to you. You’ll be able to swap from English to Spanish and again to Japanese. It is going to know what it’s doing all over – it’s phenomenal. Smaller and cheaper fashions don’t have that many languages.
The final situation is privateness. We’re built-in with IBM’s watsonx. It permits clients to make use of LLMs which are off the grid, both as a result of they run it themselves on their very own hosted setting or as a result of they use it by means of Pyramid’s internet hosting. What’s extra, Pyramid is just not sniffing your knowledge. Nothing is shared. We’re on the opposite facet of the identical location.
Privateness is the primary concern for a lot of companies relating to LLM. In actual firm deployments, corporations attempt to learn how safe the interplay with the LLM is – not simply the information however the query itself. No CTO desires to seek out that their accountant by chance typed in top-secret gross margin info of their query. It might not even be all the knowledge, only a fragment of it.
Consider the privateness situation in AI this manner – you solely need your workers to ask actual enterprise questions for actual knowledge. The extra actual and problematic the dataset is, the extra prime secret the information is. Good questions/issues and secrecy/confidentiality go hand in hand collectively.
Whenever you ask the LLM a query, you must really feel assured there’s nobody listening and that it’s only for you – nobody else is taking possession of that. Such understood privateness because it stands as we speak is a extremely sought-after operate. I imagine that there’s numerous reluctance to get going with LLMs due to that single situation.
You additionally must determine to what diploma you need to unleash AI in your customers. For instance, chances are you’ll need to have sure datasets and knowledge fashions that aren’t gen AI-enabled since you really feel involved about your customers. You’re feeling prefer it may produce the improper outcomes.
On a associated observe, you must determine the way you’re going to onboard new workers. Fortunately, the gen AI stuff is comparatively simple to make use of – that’s the entire level. However it doesn’t exist in a vacuum; it exists within the context of many capabilities and options.
To sum up, the elements for a CTO implementing Gen AI into BI are choosing the proper LLM, language, privateness, and adapting the answer to their group.
In preparation for all this, what in-house capabilities ought to a CTO foster? Some imagine cooperating with a third-party vendor skilled in AI could also be a great way for a CTO to acquire that further experience – by means of workshops and day-to-day work. What do you consider that?
The very first thing to acknowledge is that the next-generation AI interface, with all its magic, is reliant on high quality knowledge, an issue that has existed within the BI area since time immemorial.
The issue is illustrated by the “rubbish in, rubbish out” idea. If a pie chart is predicated on an incorrect or muddled database, then regardless of the pie chart says is itself muddled.
Gen AI itself may match very properly, however is underpinned by the standard of knowledge, the way in which it’s structured, and, subsequently, the semantic layer that sits between the generative engine and the information. The semantic layer can also be solely pretty much as good as the information construction.
It might sound loopy to scale back the implementation of AI to one thing as uninteresting as primary knowledge, however numerous the opposite items have been coated fairly properly and work out of the field. What kills everyone, together with Pyramid, is a poorly designed database or semantic layer.
Knowledge can also be the realm the place third events have the largest function to play. That is additionally the normal area for third events within the BI area. The problem is to return in and design a well-constructed and high-performing knowledge property that takes full benefit of its know-how stack. One other is to construct a semantic layer that matches up with the way in which enterprise customers need to devour knowledge. If they’ll try this, then gen AI will fall into place.
So it’s all in regards to the knowledge. However there’s additionally a query of the way to measure how properly you’re doing it – the definition of performed. How can a company ensure that they’re heading in the right direction – one or two years down the highway?
The very first thing you may measure is consumer adoption. You need to learn how many customers log in over a sure interval and the way they use the options and the information themselves.
Person adoption is a comparatively easy metric to seize and it’ll offer you an concept of whether or not the information is used finally to drive a call. For those who present a selected pie chart, discover out if customers take a look at it and get some insights they’ll act on.
You too can measure the variety of report requests that your builders get requested to resolve. If it goes down, you understand that your much less technical customers get solutions to extra questions extra typically with out having to go to somebody additional up the meals chain to get them the reply.
For those who develop BI self-service know-how, you will note that troublesome questions require a extra expert consumer to articulate or resolve a given pie chart and its logic. And if that consumer is utilized much less and fewer over time, which might be measured in hours or quite a few requests, you’ll know that the gen AI answer is starting to shut the hole between refined problem-solving and simplified UX.
To sum it up, I’d focus totally on measuring consumer adoption and the way busy your most technical customers are with serving to different customers get probably the most out of the answer. Another metrics price wanting into are how typically the AI instrument is opened and used or which database is used most.
Common recommendation
Let’s put all of it collectively. What would you advise CTOs and organizations which have but to embrace AI for his or her Enterprise Intelligence options however are within the strategy of studying extra about it?
You need to know that the present technology of AI for BI is a quantum leap in comparison with the whole lot that existed earlier than. For those who’re searching for a option to decrease the price of doing analytics to drive data-driven selections, now’s the time to become involved and benefit from it.
Primarily based on what I already stated, you also needs to know there are fairly good solutions throughout the ABI area to the issues of knowledge safety, efficiency, and value administration.
For those who select to sit down the present AI-driven revolution out, it would make your much less technical and non-technical customers unable to prime into the wealth of data saved in your knowledge. That may be a potential aggressive drawback that you just’re not addressing. And it’s possible your rivals are. We’re at an inflection level now that in case you don’t benefit from it, you may be lacking one thing dramatic.
Once more, I can’t stress it sufficient – the present gen AI is rather more than simply an incremental enchancment from the final period or technology of AI. It truly is a quantum leap.
Sources
Thanks, Avi, on your insights. Let’s shut it with some suggestions for studying assets. The place can managers be taught much more about AI-driven Enterprise Intelligence?
I get an infinite quantity of data from LinkedIn teams – excess of you will get on Twitter. LinkedIn provides you an expert overlay round very particular consumer teams.
You’ll be able to go straight to the analysts. In the mean time, Gartner is approach forward on this regard. Two years in the past, they realized that AI was the following gigantic tip of the spear. They’ve a wealth of information about it. I don’t agree with the whole lot they are saying, however they can provide numerous steerage to individuals who need to discover options within the BI area for AI.
Clearly, Gartner is just not free-for-all. It’s a must to purchase a subscription. But when the AI experience is related to your group, it’s properly price shopping for. They’ve performed numerous analysis on it for a few years. Many massive corporations have already gotten a subscription.
AI for Enterprise Intelligence – what’s subsequent? 4 actions for CTOs to take
Controlling your knowledge like Captain Kirk controls his ship, conversing together with your analytics engine like with the perfect buddy – Will probably be wonderful to look at the place the long run tendencies will take AI-based BI. You might be part of that progressing revolution. Simply:
- proceed to work on the high quality of your historic knowledge – that’s the place all of it begins,
- decide an answer that takes benefit of the most recent and greatest in LLM know-how,
- take into consideration the way you need to undertake an LLM-based answer to your group’s wants – chances are you’ll want to start out small,
- measure the impression of predictive analytics, knowledge visualization, and gen AI-driven BI proper from the beginning and alter accordingly.
Persist, and maybe quickly, you will see your self on the forefront of one thing actually particular.
Do you need to be taught extra about how Pyramid Analytics adjustments the world of Enterprise Intelligence with AI?
Try the official web site for knowledgeable content material, together with articles and webinars.