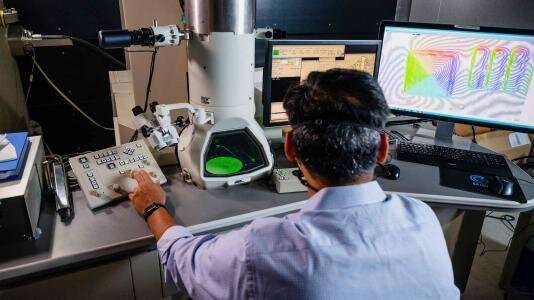
With decision 1,000 occasions higher than a lightweight microscope, electron microscopes are exceptionally good at imaging supplies and detailing their properties. However like all applied sciences, they’ve some limitations.
To beat these limitations, scientists have historically centered on upgrading {hardware}, which is expensive. However researchers on the U.S. Division of Power’s (DOE) Argonne Nationwide Laboratory are displaying that superior software program developments can push their efficiency additional.
Argonne researchers have not too long ago uncovered a approach to enhance the decision and sensitivity of an electron microscope by utilizing a synthetic intelligence (AI) framework in a singular approach. Their strategy, revealed in npj Computational Supplies, permits scientists to get much more detailed details about supplies and the microscope itself, which might additional broaden its makes use of.
“Our methodology helps enhance the decision of present devices so folks need not improve to new costly {hardware} so usually,” stated Argonne assistant scientist and lead writer Tao Zhou.
Challenges with electron microscopy as we speak
Electrons act like waves once they journey, and electron microscopes exploit this data to create photos. Photos are shaped when a cloth is uncovered to a beam of electron waves. Passing by, these waves work together with the fabric, and this interplay is captured by a detector and measured. These measurements are used to assemble a magnified picture.
Together with creating magnified photos, electron microscopes additionally seize details about materials properties, similar to magnetization and electrostatic potential, which is the power wanted to maneuver a cost towards an electrical subject. This data is saved in a property of the electron wave referred to as part. Part describes the placement or timing of some extent inside a wave cycle, similar to the purpose the place a wave reaches its peak.
When measurements are taken, details about the part is seemingly misplaced. In consequence, scientists can not entry details about magnetization or electrostatic potential from the photographs they purchase.
“Understanding these traits is important to controlling and engineering desired properties in supplies for batteries, electronics and different gadgets. That is why retrieving part data is necessary,” stated Argonne materials scientist and group chief Charudatta Phatak, a co-author of the paper.
Utilizing an AI framework to retrieve part data
Retrieving part data is a decades-old drawback. It originated in X-ray imaging and is now shared by different fields, together with electron microscopy. To resolve this drawback, Phatak, Zhou and Argonne computational scientist and group chief Mathew Cherukara suggest leveraging instruments constructed to coach deep neural networks, a type of AI.
Neural networks are primarily a collection of algorithms designed to imitate the human mind and nervous system. When given a collection of inputs and output, these algorithms search to map out the connection between the 2. However to do that precisely, neural networks must be educated. That is the place coaching algorithms come into play.
“Tech corporations like Google and Fb have developed packages of software program which might be designed to coach neural networks. What we have primarily completed is taken these and utilized them to the scientific problem of part retrieval,” stated Cherukara.
Utilizing these coaching algorithms, the analysis staff demonstrated a solution to get well part data. However what makes their strategy distinctive is that it additionally permits scientists to retrieve important details about their electron microscope.
“Usually if you’re making an attempt to retrieve the part, you presume your microscope parameters completely. Nevertheless, that data may not be correct,” Zhou identified. “With our methodology, you do not have to depend on this assumption. As a substitute, you truly get the situations of your microscope—that is one thing different part retrieval strategies cannot do.”
Their methodology additionally improves the decision and sensitivity of present tools. Which means that researchers will be capable of get well tiny shifts in part, and in flip, get details about small modifications in magnetization and electrostatic potential, all with out requiring expensive {hardware} upgrades.
“Simply doing a software program improve we had been in a position to enhance the spatial decision, accuracy and sensitivity of our microscopy,” stated Zhou. “The truth that we did not want so as to add any new tools to leverage these advantages is a large benefit from an experimentalist’s standpoint.”
The paper, titled “Differential programming enabled purposeful imaging with Lorentz transmission electron microscopy,” was revealed Sept. 6.
Growth of a high-energy-resolution, lanthanum hexaboride nanowire-based subject emission gun
Tao Zhou et al, Differential programming enabled purposeful imaging with Lorentz transmission electron microscopy, npj Computational Supplies (2021). DOI: 10.1038/s41524-021-00600-x
Argonne Nationwide Laboratory
Quotation:
Synthetic intelligence magnifies the utility of electron microscopes (2021, December 17)
retrieved 22 December 2021
from https://techxplore.com/information/2021-12-artificial-intelligence-magnifies-electron-microscopes.html
This doc is topic to copyright. Aside from any honest dealing for the aim of personal examine or analysis, no
half could also be reproduced with out the written permission. The content material is offered for data functions solely.