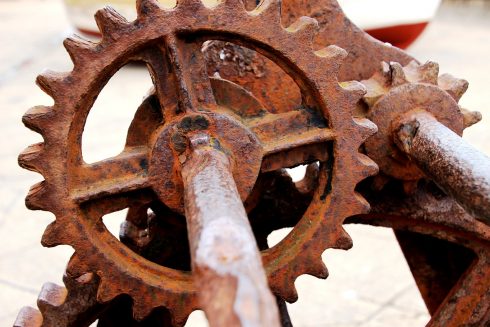
Machine Studying is rapidly changing into an vital software for automation, however failing fashions and improper background information are creating extra points than they’re fixing.
“I believe to construct an excellent machine studying mannequin… should you’re making an attempt to do it repeatedly, you want nice expertise, you want an excellent analysis course of, after which lastly you want know-how and tooling that’s form of updated and fashionable,” stated Matthew Granade, co-founder of machine studying platform supplier Domino Knowledge Lab. He defined how all three of those components have to come back collectively and function in unity in an effort to create the very best mannequin, although Granade positioned a particular emphasis on the second side. “The analysis course of determines the way you’re going to establish issues to work on, discover knowledge, work with different components of the enterprise, check your outcomes, and ship these outcomes to the enterprise,” he defined.
In line with Granade, the absence of the important mixture of these facets is the explanation why so many organizations are confronted with failing fashions. “Firms have actually excessive expectations for what knowledge science can do however they’re struggling to convey these three completely different substances collectively,” he stated. This raises the query: why are organizations investing a lot into machine studying fashions however failing to put money into the issues that can really make their fashions an final success? In line with a research performed by Domino Knowledge Lab, 97% of these polled say knowledge science is essential to long-term success, nevertheless, almost as many say that organizations lack the workers, expertise, and instruments wanted to maintain that success.
Granade traces this drawback again to the tendency to search for shortcuts. “I believe the error numerous firms make is that they form of search for a fast repair,” he started, “They search for some extent answer, or this concept of ‘I’m going to rent three or 4 actually sensible PHDs and that’s going to unravel my drawback.’ ’’ In line with Granade, these kinds of fast fixes by no means work long-term as a result of the problems run deeper. It’s all the time going to be vital to have the very best minds in your crew however they can not exist independently. With out the very best processes and finest tech to again them up, it turns into a futile try to make the most of knowledge science.
Domino Knowledge Lab’s research additionally revealed that 82% of executives polled stated they thought that management wanted to be involved about dangerous or failing fashions as the results of these fashions may very well be astronomical. “These fashions may result in dangerous choices that produce misplaced income, it may result in dangerous key efficiency indicators, and safety dangers,” Granade defined.
Granade predicts that these firms that discover themselves behind the curve on knowledge science and machine studying practices will work rapidly to appropriate their errors. Organizations which have tried and did not implement this type of know-how will maintain their eye on the others which have succeeded and take ideas the place they’ll get them. Not adapting to this follow isn’t an possibility in most industries as it is going to inevitably result in sure firms falling behind as a enterprise. Granade goes again to a complete method as the important thing to treatment the errors he has seen. “I believe you may say ‘we’re going to speculate as an organization to construct out this functionality holistically. ‘We’re going to rent the best individuals, we’re going to place a knowledge science course of in place, and the best tooling to help that course of and people individuals,’ and I believe should you do that you could see nice outcomes,” he stated.
Jason Knight, co-founder and CPO at OctoML, believes that one other side of making a profitable knowledge science and machine studying mannequin is a agency understanding of the information you’re working with. “You may assume you’ve got the best knowledge however due to underlying points with the way it’s collected or annotated or generated within the first place, it will probably form of create issues the place you may’t generate a mannequin out of it,” he defined. When there is a matter with the information that goes into producing a profitable mannequin, it doesn’t matter what approach a company makes use of, it won’t work in the best way it was meant. This is the reason it’s so vital to not skip steps when working with this type of know-how, assuming that the supply knowledge will work with out correctly understanding its particulars will spark points down the road.
Vaibhav Nivargi, founder and CTO of the cloud-based AI platform Moveworks, additionally emphasised good knowledge as being a necessary side of making a profitable mannequin. “It requires every thing from the best knowledge to characterize the actual world, to the best understanding of this knowledge for a given area, to the best algorithm for making predictions,” he stated. The mix of those will assist to make sure that the information going into creating the mannequin is reliable and can create the specified outcomes.
OctoML’s Knight additionally stated that whereas sure organizations haven’t seen the success that they had initially meant with knowledge science and machine studying, he thinks the long run is vivid. “When it comes to the long run, I stay optimistic that individuals are pushing ahead the enhancements wanted to offer options for the issues we have now seen,” he stated.